In a normal world, there are people who study deviations beyond the standard. There’s a conventional heuristic (rule of thumb) that our most significant interests fall within three standard deviations from the meanest of any measure.
I journaled here of a corporate presentation I titled ‘To 3σ and Beyond’. That, together with the opening paragraph today, are (bad) statistics-based jokes intended to refer to new learnings that may lurk within less than 6.7% of a range of products or data.
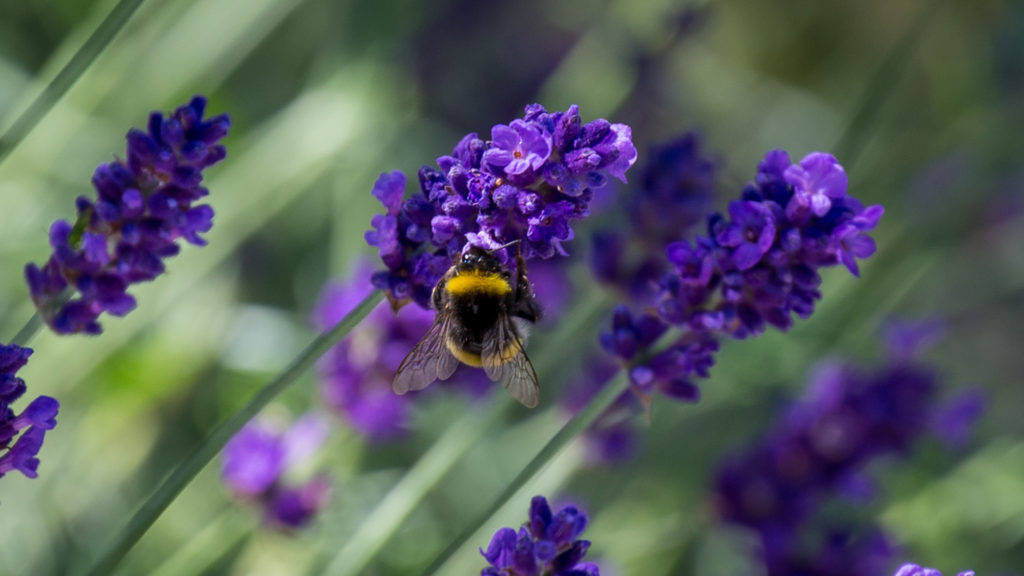
Sigma, often abbreviated as σ, is a term that comes from statistical process modelling. Sigma is a measure of standard deviation, itself a number that expresses variance within a group relative to the mean value of all measures.
I had a quick look at the Central Statistics Office in Ireland for a simple way to express the concept. The public information they produce is in the form of messages rather than methods. For example, on the issue of crime and victimisation in 2019, they say 5% of bicycle users were victims of theft. You have to interpret the infographics to understand that the likelihood of your bike being stolen is 1 in 20. So, if you own a bike, lock it up because there’s a big chance it will otherwise be nicked. The probability your bike will be stolen is approximately in the range of a 3σ event.
Sigma is about probability. Statistically speaking, if you make a million widgets, you would doubtless desire to control the process to prevent defects. In the real world, you will accept the probability that some defects will nonetheless occur. So how do you measure your effort at reducing defects?
Statistics is one very powerful measure.
‘Lean’ is a term that often summarises something that is within so called six sigma limits. A widget made within +/-6σ from the mean in a control chart is considered well controlled. In other words, there is little deviation from the standard and is within tolerance. Lean manufacturing.
Three standard deviations is 93.3%. This probably sounds like gobbledegook if you don’t know about Shewhart charts and the like. If you intend to read further, just remember that 3-Sigma in business usually connotes things that operate efficiently to ensure the highest quality products. In other words, it predicts that 6.7% of the widgets you produce will be defective. My proposition was related to typical geoscience-type data which deals tolerably well with known unknowns all the time. I believed that there were geoscience lessons hiding in what I liked to call the 3-Sigma space.
It’s different in manufacturing or health processes, for example. A probability of 3-Sigma would mean you’d expect 66,807 widgets to be defective in every million you made. Clearly that’s not good enough for a consumer who is considering buying an aeroplane or a car, defect dependent. Of course, a flaw in a car’s paintwork is less critical than a leak in the hydraulics of the braking system. So you have to prioritise.
If you, consumer or producer, survived to learn from your mistakes you could improve to 4-Sigma; there’d still be 6,201 bad widgets in a million. Remember that pilots say a good landing is one you can walk away from whereas a great landing is one where you can re-use the plane. An Airbus A320 can be rated for about 100,000 take-off-landing cycles. So 4-Sigma isn’t suitable for thrust or lift related items. You’d need to take care with weight and drag too.
Further lessons might take you to 5-Sigma (233) or 6-Sigma (3.4 defects per million widgets) and beyond. 6-Sigma is a commonly used phrase and program name in the automotive industry where it covers the spectrum from initial design to scheduled maintenance.
For this journal, I’m looking at it the other way around. I’m looking at the people rather than the products. I thought it might help to introduce the products first, to help put the scale into perspective.
I’m seeking to draw attention to the exceptional end of the distribution rather than defects. I’m wondering where the exceptional people lurk in ‘sigma’ space. Probabilistically speaking, there must be as many exceptional thinkers as there are dullards. I want to know how many more Marie Curies can be expected in a generation.
A friend of mine once told me of a welcome-type family-professor meeting he attended in an Oxbridge college. The maths professor welcomed a batch of new college entrants to his department reminding the happy dozen or so, and the proud parents of these persistent over-achievers, that they were all the same and that they should get used to being average performers. He told them the adjustment to the college environment after eighteen years of being exceptional would be hard for most of them. Note the encouraging word ‘most’ and the common misuse of ‘exceptional’. Exceptional ability is not easily assessed in a class size of a dozen nor a thousand.
Some of my colleagues at my former employer talked of our recruitment policies as targeting exceptional graduates. I preferred other corporatisms such as attracting high performers. Our incoming masters and doctors typically struggled to adjust to the corporation’s annual performance grading system. There were only three grades. The system was designed such that approximately 98% of the employees would meet the expectations they set for themselves for a year’s work. The ambitious new hires often felt insecure. They wanted to become the one in a hundred that might exceed annual expectations if they were going to stand out from their peers. We traded on their insecurity. Many were self-motivated and worked doubly hard in order to stand-out.
I should say that a few of the highest performers clashed with the management that wished to retain their skills in an ultra-competitive market. I thought (and still think) it was impossible that anyone could always exceed expectations unless they were allowed to set annual goals within their comfort zones. It was a rare case where single employee bargaining power had the edge over the employer.
Was Marie Curie a high performer? Yes, focussed and obsessive, she did things never done before. Was she 6-Sigma?
What about Isaac Newton? He was good at maths. Many say he was exceptional.
Was he 6-Sigma? In fact, he stood out from 800 million people, which was the global population at the time. So he was more like 7-Sigma, closer to one in a billion. Or was he? What about Leibnitz?
I’ll bet Netwton would have stood out from any dozen incoming students. I’ll bet he would have been graded as exceeding expectations most years.
The thing about the university professor and my former employer is that women were included. The thing about Newton’s period is that women were not.
Gallileo. Halley. Bernoulli. Hooke. Huygens.
Vermeer. Rembrandt.. El Greco.
Swift. Bacon. Milton. Pepys.
Virginia Woolf looked at the lack of opportunity for women in 1929. A Room of One’s Own is an extended essay that I just reread. She illustrated her observations with Judith, a fictional sister for William Shakespeare. Judith stayed at home while her brothers went off to school. This was what happened to Woolf. A marriage is arranged for Judith so she flees to London to become an actor. Her agent gets her pregnant and all opportunity evaporates and she kills herself. A private room and personal money are the symbols Woolf uses to represent the countless inequalities between men and women. Almost a century has passed, there are now four times as many women under the yoke as there were in 1929.
Marie Curie was one who bucked the trend. Was she alone? No, but was she very rare? Yes. Woolf too.
Newton was one in 800 million. If we assume the gender bias prevented a woman of the same intellect having the same opportunities, then Newton was one of two. Perhaps you’d agree he was one in 400 million.
The other thing is that modern university and corporate policies are no longer focussed on white, European males.
Newton was in Europe, which had about a quarter of the world’s population at the time. Let’s assume he had a peer in each of those other places so now he’s one of 100 million.
Critically, not everyone in Europe had access to the quality of life, health and education that enabled Newton to flourish. Some estimates suggest that 99% of the world had limited access to these benefits. Which means Newton was maybe one in a million after all.
I’ve broadly massaged the numbers to tell a story that I hope will help you hear my thoughts.
If you thought Newton was one in 800 million in his day, then today, the population being a magnitude larger, there should be 10 Newtons out there.
If Newton was one in a million because of his circumstances, opportunities that were not available to others who might have developed into exceptions, there should be great value in educating, feeding and looking after 8 billion in order to benefit from the intellects of 8000 Newtons.
Imagine how the world could progress with 8000 Newtons, 8000 Shakespeares, 8000 Bachs and 8000 Vermeers. Or 8000 Curies, assuming gender is no longer a barrier.
Who was Lisa Meitner? Rosalind Franklin? Jocelyn Bell Burnell? Barbara McClintock? Dorothy Hodgkin? Have a read about the good but slow work of the Athena Forum which tries to inform the wider debate on diversity in Science, Technology, Engineering, Mathematics and Medicine.
I know that none of this makes literal sense. I just want to point out some inadequacies. Bernard Mandeville had a view that you need the poor people to make society work. His poem The Grumbling Hive was published in 1705 and had a massive influence on Voltaire and especially on Rousseau. He used a beehive as a metaphor for society and in the course of the poem, he shows us how bad the analogy is while positing a transition from utopian cooperative behaviour to dystopian independent action. Two paradises? China and America in 2020 for example?
‘Vast Numbers thronged the fruitful Hive;
Yet those vast Numbers made ’em thrive;’
Eventually becomes:
‘Thus every part was full of vice!
But the whole mass a paradise.’
There’s an interesting insight into the challenges the world faces on Dollar Street (from Gapminder and the Hans Rosling family). Look at the TEDx talk here too.
My narrative might not stand the scrutiny of a male statistician but perhaps a female might see the message beyond my inaccurate numbers. It was Woolf who wrote ‘The history of men’s opposition to women’s emancipation is more interesting perhaps than the story of that emancipation itself’. I was thinking about the military tactic of siege and how old ideas are so resilient. Perhaps a usurper must, and will, starve others of sustenance in order to foment change. Figuratively speaking.
I invite corrections and clarifications in the hope that it fosters thought and discourse on the unconscionable inequality that limits the ability of 8 billion people to look after themselves and one another properly.
Is it possible to set a ‘lean’ education goal and create a system that educates (almost) everyone?
Leave a Reply